Por favor, use este identificador para citar o enlazar este ítem:
http://hdl.handle.net/20.500.14076/17949
Título : | Minería de datos aplicación a la fiabilidad crediticia |
Autor : | Venegas Palacios, Edgard Kenny Kahn Casapía, Joseph Luis |
Palabras clave : | Minería de datos;Métodos bayesiano;Redes neuronales artificiales |
Fecha de publicación : | dic-2015 |
Editorial : | Universidad Nacional de Ingeniería |
Citación : | Venegas Palacios, E. & Kahn Casapía, J. (2015). Minería de datos aplicación a la fiabilidad crediticia. REVCIUNI, 18(1). |
Citación : | Volumen;18 Número;1 |
Resumen : | Los modelos de clasificación en Minería de Datos, enfocados a predecir si un cliente de un banco es fiable o no para recibir un crédito, presentan un score y precisión con entrenamiento simple para RL: 0.21% y 0.79%, NB: 0.74% y 0.76%, AD: 0.70% y 0.69%, RNA: 0.74% y 0.73%, con entrenamiento cruzado para R.L: 0.20% y 0.79%, NB: 0.73% y 0.72%, AD: 0.68% y 0.72%, RNA: 0.73% y 0.75%. Además de utilizar RL para encontrar relaciones entre las variables de la base de datos disponible. Classification models in Data Mining, focused on predicting whether a customer of a bank is reliable or not for credit, have a score and accuracy with simple training for RL: 0.21% and 0.79%, NB: 0.74% and 0.76%, AD: 0.70% and 0.69%, RNA: 0.74% and 0.73%, cross training RL: 0.20% and 0.79%, NB: 0.73% and 0.72%, AD: 0.68% and 0.72%, RNA: 0.73% and 0.75%. In addition to using RL to find relationships between variables of the database available. |
URI : | http://hdl.handle.net/20.500.14076/17949 |
ISSN : | 1813 – 3894 |
Correo electrónico : | kenny@imca.edu.pe jkahn@uni.pe |
Derechos: | info:eu-repo/semantics/restrictedAccess |
Aparece en las colecciones: | Vol. 18 Núm. 1 (2015) |
Ficheros en este ítem:
Fichero | Descripción | Tamaño | Formato | |
---|---|---|---|---|
REVCIUNI_Vol18-n1-Art.7.pdf | 1,09 MB | Adobe PDF | Visualizar/Abrir |
Este ítem está sujeto a una licencia Creative Commons Licencia Creative Commons
Indexado por:
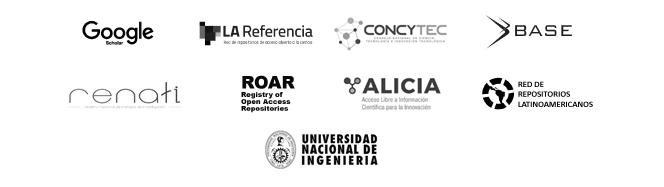