Por favor, use este identificador para citar o enlazar este ítem:
http://hdl.handle.net/20.500.14076/19062
Título : | Estimación de parámetros geométricos musculoesqueléticos y del tejido celular subcutáneo en infantes menores a 5 años usando imágenes de ultrasonido |
Autor : | Erquinigo Agurto, Ronal Arturo |
Asesor : | Román González, Avid |
Palabras clave : | Redes neuronales;Antropometría;Imágenes de ultrasonido |
Fecha de publicación : | 2019 |
Editorial : | Universidad Nacional de Ingeniería |
Resumen : | Estimar el peso y la talla en pacientes inmovilizados, es una alternativa ante la dificultad técnica de pesar y tallar a los mismos. En literatura, se han propuesto diversos métodos que, basados en la antropometría del paciente, permiten estimar el peso, así como la talla en menor medida, con un mínimo de error posible. Mediciones específicas tales como la circunferencia y la longitud del brazo son utilizadas en modelos previamente armados a partir de poblaciones heterogéneas. Tal es el caso de la cinta Mercy, la cual fue desarrollada a partir de la base de datos NHANES (National Health and Nutrition Examination Survey) y que demostró un menor error de estimación sobre otros métodos; a pesar de ello, su uso viene siendo validado en otras poblaciones geográficas. Por otro lado, el uso de imágenes de ultrasonido para la extracción automática de parámetros geométricos musculoesqueléticos (PGM) ha sido poco estudiado y limitado a ciertas patologías debido a los artefactos, a su bajo nivel de señal a ruido y a que sus superficies óseas aparecen con un grosor mayor que dificulta el contraste entre el hueso y el músculo circundante. Sin embargo, el uso de técnicas de procesamiento digital de imágenes y redes neuronales permite que su uso pueda ser extendido a otras aplicaciones adicionales tal como la estimación del peso y la talla a partir de los PGM extraídos.
El presente trabajo consiste en dos partes. La primera parte es una prueba de concepto que tiene como objetivos: a) la recolección de una base de datos principal de 43 niños menores de 5 años, cuyos datos obtenidos fueron la talla, el peso, la edad, el sexo y los PGM estimados manualmente a través de imágenes de ultrasonido del brazo (diámetro y profundidad del húmero), el muslo (diámetro y profundidad del fémur) y el pecho (perímetro, área y profundidad de la costilla), y b) generar dos modelos estadísticos para estimar el peso y la talla en función de las demás variables, para la cual, se utiliza el método de regresión lineal múltiple que, a partir de los métodos incremental y saturado, identifica aquellos PGM que, como predictores, tienen una significancia estadística mayor al 95%. La segunda desarrolla un algoritmo que permite extraer automáticamente información geométrica sobre imágenes de ultrasonido del fémur, específicamente, los PGM correspondientes a la profundidad y el diámetro del fémur, que demostraron ser los mejores predictores para estimar el peso y la talla según la prueba de concepto, cuyo método desarrollado consiste en las siguientes etapas, que son: el preprocesamiento mediante la cual se resalta las estructuras óseas, así como otras estructuras musculares, la umbralización global y la segmentación y extracción de características de la región de interés correspondiente posiblemente a la superficie ósea del fémur. Después de validar la región de interés utilizando una red neuronal, previamente entrenada, se identifica si la región segmentada corresponde a la superficie del fémur o no, y se procede a la estimación de la profundidad y del diámetro del fémur.
Los resultados obtenidos para la prueba de concepto muestran un coeficiente de correlación ajustado al cuadrado de 0.8438 y 0.8726 para los modelos de estimación del peso y la talla, respectivamente. El algoritmo de extracción automática de PGM del fémur, consiguió errores relativos promedio de 2.69% y 7.58% para las estimaciones manuales de profundidad y diámetro del fémur, respectivamente. El estimador, que utiliza los modelos de estimación a partir de los PGM extraídos automáticamente, estima con un error relativo menor del 10%, para una población de 38 niños, el peso en el 63.16% de los casos y la talla en el 89.47% de los casos. Estimating weight and height in immobilized patients is an alternative to the technical difficulty of measuring their weight and height. In the literature, there have been proposed various methods that, based on the anthropometry of the patient, allow the estimation of weight, as well as height to a lesser extent, with a minimum possible error. These methods are based on the anthropometry of the patient. Specific measurements such as the mid upper arm circumference (MUAC) and upper arm length are used in models developed from heterogeneous populations. Such is the case of the Mercy tape, which was developed using the NHANES database (National Health and Nutrition Survey) and which shows a lower estimation error than other methods. Despite this, its use is being validated in other geographical populations. On the other hand, the use of ultrasound for the automatic extraction of musculoskeletal geometric parameters (MGP) has been little studied and limited to certain pathologies due to the artifacts, the low signal to noise ratio and that bone boundaries appear with a greater thickness that makes it difficult the contrast between the bone and the surrounding muscle. However, the use of digital image processing techniques and neural networks allows their use to be extended to other additional applications such as the estimation of weight and height from the extracted MGP. The present work consists of two parts. The first one is a proof of concept which objectives are the collection of a main database of 43 children under 5 years old. The data obtained were height, weight, age, sex, and the manually obtained MGP through ultrasound images of the arm (diameter and depth of the humerus), thigh (diameter and depth of the femur) and chest (perimeter, area, and depth of rib). The next objective is to generate a statistical model to estimate weight and height that use as predictors these variables. For this purpose, a multiple linear regression model is used that, based on the forward stepwose and backward elimination methods, identifies those MGP that, as predictors, have a statistical significance greater than 95%. The second part aims to develop an algorithm that automatically extracts geometric information from ultrasound images of the femur. Specifically, the MGP corresponding to the depth and diameter of the femur, which proved to be the best predictors for estimating weight and height according to the proof of concept. The method developed consists, first of all, in a preprocessing stage by means of which the bony structures are enhanced, as well as other muscular structures; then a global thresholding step is applied, followed by a segmentation stage and the feature extraction from the region of interest likely corresponding to the bony surface of the femur. After validating the region of interest with a previously trained neural network, which allows identifying if the segmented region corresponds to the femur surface or not, the femur depth and diameter are estimated. The results obtained for the proof of concept show an adjusted squared correlation coefficient of 0.8438 and 0.8726 for the weight and height estimation models respectively. For the algorithm of automatic extraction of MGP from the femur, average relative errors of 2.69% and 7.58% were obtained regarding to the manual estimates for depth and diameter of the femur respectively. The estimator, that uses the estimation models and the automatically extracted MGP as input, estimates within a relative error of less than 10%, for a population of 38 children, the weight in 63.16% of the cases and the height in 89.47% of the cases. |
URI : | http://hdl.handle.net/20.500.14076/19062 |
Derechos: | info:eu-repo/semantics/openAccess |
Aparece en las colecciones: | Maestría |
Ficheros en este ítem:
Fichero | Descripción | Tamaño | Formato | |
---|---|---|---|---|
erquinigo_ar.pdf | 3,1 MB | Adobe PDF | Visualizar/Abrir |
Este ítem está sujeto a una licencia Creative Commons Licencia Creative Commons
Indexado por:
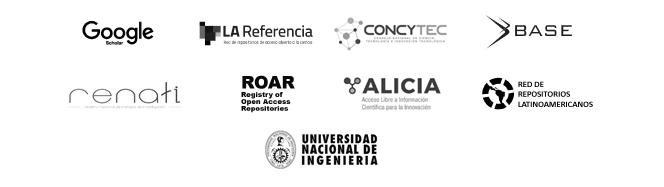