Por favor, use este identificador para citar o enlazar este ítem:
http://hdl.handle.net/20.500.14076/12554
Título : | Minería de opiniones subjetivas aplicado a una red social de microblogging usando técnicas de minería de textos y máquina vector soporte |
Autor : | Audante Ramos, Néstor Rafael Huamaní Montesinos, Enrique |
Asesor : | Oporto Díaz, Samuel Alonso |
Palabras clave : | Aprendizaje automático;Clasificación de textos;Máquina vector soporte (SVM) |
Fecha de publicación : | 2017 |
Editorial : | Universidad Nacional de Ingeniería |
Resumen : | Esta investigación se orienta a la detección automática de la polaridad de sentencias subjetivas (es decir, en la detección de si una frase emitida en un medio, con respecto a un ente específico, es positiva o negativa), usando como fuente de análisis la red social virtual de microblogging: Twitter. En este estudio se ha considerado que la opinión de un tweet está compuesta por la síntesis de la opinión del texto del tweet y de la url que tiene adjunta.
Detectar la polaridad de sentencias típicamente presenta 2 tareas:
1. Detectar si una setencia es objetiva o subjetiva, tarea conocida como clasificación de subjetividad, y que requirió desarrollar un Procedimiento de asignación de categoría subjetiva a sentencias de Twitter (basado en reglas y pistas de subjetividad) y dos clasificadores supervisados, basados en SVM (para el texto del tweet y el texto de su url respectivamente)
2. Detectar la polaridad de la sentencia subjetiva, tarea conocida como clasificación de Sentimiento, y que requirió desarrollar dos clasificadores supervisados, basados en SVM (para el texto del tweet y el texto de su url respectivamente). Estos clasificadores indicarán si la sentencia que analizan presenta una opinión positiva o negativa.
La metodología usada en esta investigación es CRISP-DM. Para la construcción del modelo se generó (1) un conjunto de 441,717 tweets anotados mediante etiquetado ruidoso, así como (2) corpus de 7,948 pistas de subjetividad, (3) corpus de 10,000 artículos de periódicos con contenido objetivo y subjetivo y, (4) corpus de 3,350 reseñas de peliculas divididas entre positivas y negativas, todo en idioma español.
Luego de realizar el análisis, limpieza y transformación de los datos, se desarrolló el modelo de solución propuesto, que consta del arreglo de clasificadores supervisados mencionados anteriormente. Adicionalmente se realizó la experimentación del modelo, ejecutándose 3,840 corridas, identificándose los valores óptimos para los parámetros establecidos con anterioridad: (1) Tipo de Kernel: DOT, (2) Esquema de creación de Vector de Palabras: TF y, (3) Peso Mínimo de Ganancia de Información: 0.03. Se realizó la validación del modelo total desarrollado (con un dataset de 208 tweets, anotados a mano) y se obtuvo una exactitud total (Acurracy) del 89.2% y una precisión del 88.69%. Asimismo, se detalló las conclusiones y recomendaciones a tomar en cuenta para futuras investigaciones en los campos de análisis sentimental, minería de textos o campos afines. This research is focused on the automatic detection of the polarity of subjective sentences (i.e. detecting whether a phrase, posted on social media, about a specific entity, has positive or negative connotation), using the virtual social network microblogging site: Twitter. This study has considered that the opinion of a tweet is composed by the synthesis of the opinion of the text of the tweet and of the URL that is attached. Detecting the polarity of sentences typically consists of 2 tasks: 1. Detecting whether a sentence is objective or subjective, a task known as “Subjectivity classification”, that required the development of a procedure to assign a subjective category to Twitter sentences (based on rules and subjectivity clues) and two supervised classifiers, based on SVM (for the text of the tweet and its URL). 2. Detecting polarity of a subjective sentence, a task known as “Sentiment Classification”, that required the development of two supervised classifiers, based on SVM (for the text of the tweet and its URL). These classifiers will indicate if the sentence, analyzed, presents a positive or a negative opinion. The methodology used in this research was CRISP-DM. To build the model, (1) a dataset of 441,717 tweets, labeled by noisy labeling, was generated, as well as (2) a corpus of 7,948 subjectivity clues, (3) a corpus of 10,000 journal articles, with objective and subjective content, and (4) a corpus of 3,350 movie reviews, divided between positive and negative, all in the Spanish language. After performing the analysis, the cleaning and the data transformation tasks, the proposed solution model was developed, which consists of the array of the supervised classifiers previously mentioned. Additionally, an experimental design was conducted, which consisted of 3,840 runs, finding the optimal values for the previously tested parameters: (1) Kernel type: DOT, (2) Word vector creation scheme: TF and, (3) Minimum Weight of Information Gain: 0.03. Validation of the entire model was performed (with a 208 tweets dataset, hand-annotated) getting a total of 89.2% accuracy and 88.69% of precision. Likewise, the conclusions and recommendations to be taken into consideration for future research in the fields of sentimental analysis, text mining or related topics, were detailed. |
URI : | http://hdl.handle.net/20.500.14076/12554 |
Derechos: | info:eu-repo/semantics/restrictedAccess |
Aparece en las colecciones: | Ingeniería de Sistemas |
Ficheros en este ítem:
Fichero | Descripción | Tamaño | Formato | |
---|---|---|---|---|
audante_rn.pdf | 4,29 MB | Adobe PDF | Visualizar/Abrir |
Este ítem está sujeto a una licencia Creative Commons Licencia Creative Commons
Indexado por:
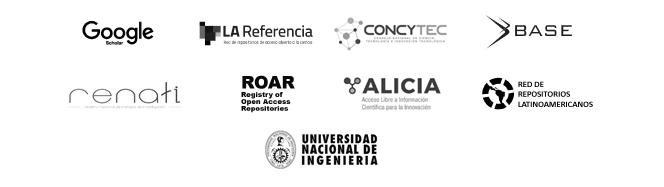