Please use this identifier to cite or link to this item:
http://hdl.handle.net/20.500.14076/13728
Title: | Efectos de la estructura estadística de los datos en la implementación de la red neuronal autosupervisada Effects of statistical data structure on the implementation of a self-supervised neural network |
Authors: | Huamanchumo De la Cuba, Luis Emilio Sánchez Alvarado, Luis Alberto |
Keywords: | Estadística;Análisis de componentes;Algoritmo hebbiano;Reducción de dimensionalidad |
Issue Date: | 1-Jun-2013 |
Publisher: | Universidad Nacional de Ingeniería |
Citation: | Huamanchumo de la Cuba, L., & Sánchez Alvarado, L. (2013). Efectos de la estructura estadística de los datos en la implementación de la red neuronal autosupervisada. TECNIA, 23(1). https://doi.org/10.21754/tecnia.v23i1.68 |
Series/Report no.: | Volumen;23 Número;1 |
Related URI: | http://revistas.uni.edu.pe/index.php/tecnia/article/view/68 |
Abstract: | La presente investigación plantea como objetivo estudiar aspectos técnicos relacionados con la implementación de la red neuronal de Análisis de Componentes Principales (ACP) en términos de su capacidad predictiva, generalización y precisión con el fin de establecer criterios óptimos para su validación, evaluación del desempeño e implementación. Para ello, se plantea la hipótesis de que la estructura estadística de los datos influye significativamente en el óptimo desempeño de la red neuronal de ACP en el contexto no supervisado. Se demostró que el algoritmo Hebbiano de la fase de aprendizaje garantiza la calidad de representación de la red debido a que capitaliza eficientemente la información en escenarios con varianza generalizada grande. The purpose of this research is to study technical aspects involved in the implementation of a Principal Component Analysis (PCA) neural network in terms of predictive capacity, generalization and accuracy in order to establish optimal criteria for the validation and implementation thereof. Our hypothesis is that the statistical structure of the data affects the optimal performance of a PCA neural network in the unsupervised context. It was demonstrated that the Hebbian algorithm at the learning phase ensures enhanced quality of network representation as it makes efficient use of information where generalized variance is large. |
URI: | http://hdl.handle.net/20.500.14076/13728 |
ISSN: | 2309-0413 |
E-mail: | lhdlc40@gmail.com |
Rights: | info:eu-repo/semantics/openAccess |
Appears in Collections: | Vol. 23 Núm. 1 (2013) |
Files in This Item:
File | Description | Size | Format | |
---|---|---|---|---|
TECNIA_Vol.23-n1-Art. 7.pdf | 197,61 kB | Adobe PDF | View/Open |
This item is licensed under a Creative Commons License
Indexado por:
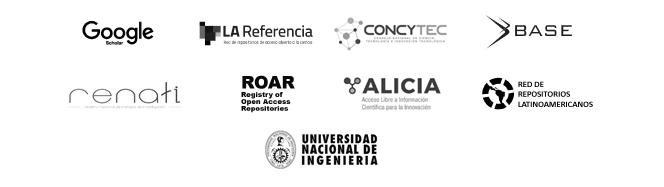