Please use this identifier to cite or link to this item:
http://hdl.handle.net/20.500.14076/18447
Title: | Forescat modeling of spatio-temporal raster data using principal component analysis and a neural networks - wavelet decomposition model |
Authors: | Amao Suxo, Christian |
Advisors: | Velásquez Castañón, Oswaldo José |
Keywords: | Predicciones;Redes neuronales;Datos ráster |
Issue Date: | 2018 |
Publisher: | Universidad Nacional de Ingeniería |
Abstract: | Nowadays spatio-temporal forecasting has been drawing more and more attention from academia researchers and industrial practitioners for its great utility to plan and develop contingency measures against future adverse conditions. In this thesis, a methodology to forecast maps in spatio- temporal raster datasets is proposed. Following a summarize-predict-and-rebuild methodology, it 1) first suggests a reduction in the dimensionality of data using a principal component analysis, then 2) individual forecasts on the most significant components or eigenvectors are calculated using a neural networks - wavelet decomposition model. Finally, 3) a recursive algorithm, applied on the spectral inverse reconstruction of the individual forecasts, provides the final forecast maps.
The devised methodology led to three models according: the spatial principal component anal¬ysis (SPCA) model, the temporal principal component analysis (TPCA) model and the spatio- temporal principal component analysis (STPCA) model. In order to evaluate their forecasting accuracy, a simulation study was carried out by considering datasets with pure temporal, pure spatial and spatio-temporal variability. The results suggest using a TPCA (or SPCA) model when the temporal (or spatial) variability is predominant. For datasets with similar spatial and temporal variability information, the STPCA model provides the best forecast results. The research culmi- nates with a real-world case study in monthly sea surface temperature anomalies of the Tropical Pacific Ocean. En la actualidad, el pronóstico de datos espacio - temporales ha sido de especial interés para investigadores académicos y profesionales de la industria por su gran utilidad para planificar y desarrollar medidas de contingencia contra futuras condiciones adversas. En este trabajo se pro¬pone una metodología para el pronóstico de mapas tipo ráster. Siguiendo una metodología de resumir-predecir-y-reconstruir, el método sugiere reducir la dimensionalidad de los datos usando un análisis de componentes principales para luego realizar pronósticos individuales sobre las com¬ponentes o auto vectores más significativos. Finalmente, un algoritmo recursivo, aplicado sobre la reconstrucción inversa espectral de los pronósticos individuales, brinda el pronóstico final de los mapas. La metodología propuesta da lugar a tres modelos: el modelo espacial de componentes princi¬pales (ECP), el modelo temporal de componentes principales (TCP) y el modelo espacio - temporal de componentes principales (ETCP). Con el fin de evaluar su capacidad de pronóstico, se real¬iza un estudio de simulación considerando datos con una estructura de variabilidad espacial pura, temporal puro y espacio - temporal. Los resultados sugieren usar un modelo TCP (o ECP) cuando la variabilidad temporal (o espacial) es predominante. Para datos con similar información en la variabilidad espacial y temporal, el modelo ETCP brinda los mejores resultados de pronóstico. El trabajo culmina con una aplicación real en datos mensuales de anomalías de temperatura superficial del mar del océano Pacífico Tropical. |
URI: | http://hdl.handle.net/20.500.14076/18447 |
Rights: | info:eu-repo/semantics/openAccess |
Appears in Collections: | Maestría |
Files in This Item:
File | Description | Size | Format | |
---|---|---|---|---|
amao_sc.pdf | 7,48 MB | Adobe PDF | View/Open |
This item is licensed under a Creative Commons License
Indexado por:
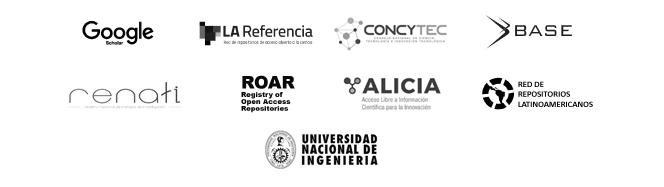