Please use this identifier to cite or link to this item:
http://hdl.handle.net/20.500.14076/19048
Title: | Redes neuronales artificiales aplicadas a la remoción de cobre en agua utilizando quitosano entrecruzado con glutaraldehído |
Authors: | Blanco Lecca, Manuel Enrique |
Advisors: | Jacinto Hernández, Christian Ronald |
Keywords: | Redes neuronales artificiales;Sistemas inteligentes;Inteligencia artificial |
Issue Date: | 2018 |
Publisher: | Universidad Nacional de Ingeniería |
Abstract: | Con el objeto de estudiar un sistema inteligente capaz de predecir el comportamiento porcentual en la remoción de cobre en aguas desionizada, se ha diseñado una técnica de inteligencia artificial aplicado al problema químico de remoción de Cu (II) en aguas, teniendo como soporte de programación el software MATLAB R2014a. Este sistema ha sido entrenado inicialmente con 74 datos ingresados como patrones de aprendizaje, teniendo como parámetros de ingreso para la red neuronal artificial 6 parámetros críticos de la experimentación: pH, masa de quitosano entrecruzado con glutaraldehído (g), concentración de glutaraldehído (GLA) (g/100mL), tiempo de agitación (min), frecuencia de agitación (rpm), y concentración inicial de cobre (ppm). Se verificó que el máximo error de predicción del modelo de red neuronal artificial del tipo perceptrón multicapa (14.15%) se obtiene a partir de 16 capas ocultas. Para comprobar la efectividad de la red neuronal, se hizo uso de 9 mediciones distintas a las de la matriz de experimentos, para así realizar la validación de este sistema, verificando así la efectividad de nuestra red neuronal (a un 98.79% de correspondencia entre los valores predictivos, respecto a los valores reales observados). La descontaminación se logra por captura del ion Cu (II) (analito de estudio en aguas) por las perlas de quitosano entrecruzado con glutaraldehído. La obtención de los datos experimentales se toma por diferencia porcentual entre la concentración inicial del agua (contaminada con CuSO4.5H2O) y la concentración obtenida después del proceso de descontaminación con las perlas de quitosano entrecruzadas con glutaraldehído, registrado por la técnica de espectrofotometría de absorción atómica. To study an intelligent system capable of predicting the percentage behavior in the removal of copper in deionized waters, an artificial intelligence technique has been designed applied to the chemical problem of metal removal (specifically Cu (II)) in waters, taking MATLAB R2014a as software for to programming support. This system has been initially trained with 74 data entered as learning patterns, having as input parameters, for the artificial neural network, 6 critical parameters of the experiment: pH, mass of chitosan cross-linked with glutaraldehyde (g), concentration of glutaraldehyde (GLA) (g / 100mL), stirring time (min), stirring frequency (rpm), and initial copper concentration (ppm). It was verified that the maximum prediction error of the artificial neural network model of the multilayer perceptron type (14.15%) is obtained from 16 hidden layers. To check the effectiveness of the neural network, 9 different measurements were used to those of the experiment matrix, in order to validate this system, thus verifying the effectiveness of our neural network (at 98.79% correspondence between the predictive values, with respect to the actual values observed). Decontamination is achieved by capturing the Cu (II) ion (analyte studied in water) by the chitosan beads cross-linked with glutaraldehyde. Obtaining the experimental data is taken as a percentage difference between the initial water concentration (contaminated with CuSO4.5H2O) and the concentration obtained after the decontamination process with the chitosan beads cross-linked with glutaraldehyde, registered by the absorption spectrophotometry technique atomic. |
URI: | http://hdl.handle.net/20.500.14076/19048 |
Rights: | info:eu-repo/semantics/openAccess |
Appears in Collections: | Maestría |
Files in This Item:
File | Description | Size | Format | |
---|---|---|---|---|
blanco_lm.pdf | 2,87 MB | Adobe PDF | View/Open |
This item is licensed under a Creative Commons License
Indexado por:
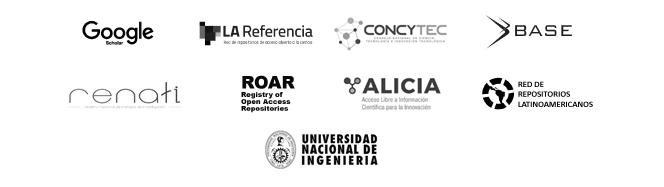