Please use this identifier to cite or link to this item:
http://hdl.handle.net/20.500.14076/22525
Title: | Machine Learning aplicado al control de la fragmentación de rocas en la voladura de minas a tajo abierto |
Authors: | Idrogo Zamora, Yessica Pamela |
Advisors: | Zambrana Flores, Henry Mamfredo |
Keywords: | Voladura de rocas;Machine learning;Mina a tajo abierto |
Issue Date: | 2022 |
Publisher: | Universidad Nacional de Ingeniería |
Abstract: | La presente tesis desarrolla el análisis de datos y modelamiento predictivo en la voladura para una mina a tajo abierto.
La primera etapa consiste en el planteamiento del problema a resolver, esto incluye identificar los objetivos, y en el caso de un modelamiento de machine learning, definir cuál o cuáles son las variables objetivo que se van a predecir, esta variable a predecir será vital para resolver el problema en cuestión. En esta etapa se identifica cuál es el algoritmo o los algoritmos que se van a utilizar para resolver el problema en cuestión.
La segunda etapa consiste en el análisis de datos, donde se analiza los tipos de variables, la cantidad de datos disponibles, las categorías que se presentan, las correlaciones entre variables, y la distribución estadística de estas variables.
La tercera etapa es el tratamiento de datos, donde se realizan transformaciones sobre las variables de la base de datos. En la presente tesis se realizó los tratamientos de estandarización para homogeneizar el rango de cada parámetro del modelo y la transformación de Yeo Johnson para normalizar las distribuciones de datos que poseen un alto sesgo.
La cuarta etapa consiste en realizar el modelo de machine learning para conseguir la variable de interés, en este caso se usaron redes neuronales artificiales, que tuvieron como variables de entrada los parámetros que ya fueron analizados y tratados en las etapas anteriores; y el output fue la variable de interés que permite resolver el problema planteado en la primera etapa.
La quinta etapa consistió en análisis de resultados donde se evaluó el impacto del programa basado en inteligencia artificial para cumplir el objetivo deseado de generar la ubicación de perforación de los holes que cumpla con el target de fragmentación; y, esto mediante una comparativa de los resultados obtenido mediante el método tradicional versus el método usando redes neuronales artificiales.
Mencionar que, la flexibilidad de la red neuronal artificial permite a los ingenieros generar modelos que se adecuen a sus necesidades de diseño, costo y objetivo de la
Fragmentación como llevar a un chancado y molienda. This thesis develops the data analysis and predictive modeling in blasting for an open pit mine. The first stage consists of posing the problem to be solved, this includes identifying the objectives, and in the case of a machine learning modeling which is the target variable or variables to be predicted, this variable to be predicted will be vital to solve the problem in question. At this stage, the algorithm or algorithms that are going to be used to solve the problem in question are identified. The second stage consists of data analysis where the types of variables, the amount of data available, the categories that are presented, the correlations between variables, and the statistical distribution of these variables are analyzed. The third stage is the data treatment where transformations are carried out on the variables of the database in the present thesis, the standardization treatments were carried out to homogenize the range of each parameter of the model and the Yeo Johnson transformation to normalize the distributions of data that are highly based. The fourth stage consists of carrying out the machine learning model to obtain the variable of interest, in this case artificial neural networks were used, which had as input variables the parameters that were already analyzed and treated in the previous stages and the output was the variable of interest that allows solving the problem posed in the first stage. The fifth stage consisted of analysis of results where the impact of the program based on artificial intelligence was evaluated to meet the desired objective of generating the drilling location of the holes that meets the fragmentation target and this through a comparison of the results obtained through the traditional method versus the method using artificial neural networks. Mention that, the flexibility of the artificial neural network allows engineers to generate models that adapt to their design needs, cost and fragmentation objective such as leading to crushing and grinding. |
URI: | http://hdl.handle.net/20.500.14076/22525 |
Rights: | info:eu-repo/semantics/openAccess |
Appears in Collections: | Ingeniería de Minas |
Files in This Item:
File | Description | Size | Format | |
---|---|---|---|---|
idrogo_zy.pdf | 2,47 MB | Adobe PDF | View/Open | |
idrogo_zy(acta).pdf | 85,87 kB | Adobe PDF | View/Open |
This item is licensed under a Creative Commons License
Indexado por:
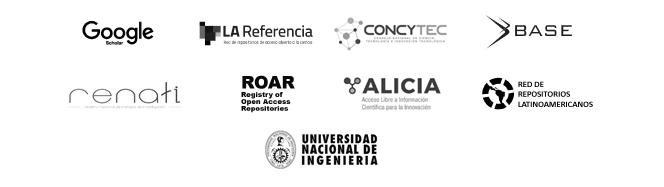