Please use this identifier to cite or link to this item:
http://hdl.handle.net/20.500.14076/26993
Title: | Análisis de imágenes hiperespectrales para la detección y cuantificación de trozos de plástico mezclados con granos y microplásticos mezclados con harina de almendra |
Authors: | Apaza Mamani, Hebbert Alexander |
Advisors: | Loro Ramírez, Héctor Raúl |
Keywords: | Microplásticos;Imágenes espectrales |
Issue Date: | 2021 |
Publisher: | Universidad Nacional de Ingeniería |
Abstract: | Se presentan imágenes hiperespectrales de plásticos mezclados con granos de almendras, lentejas, alverjas y arroz, así como de micro plásticos mezclados con harina de almendra obtenidas en el laboratorio usando una cámara hiperespectral RESONON modelo Pika NIR. La identificación y cuantificación de abundancias de plásticos dentro de un grupo de granos presentes en una imagen hiperespectral se realizó usando dos caminos o procedimientos. En el primer procedimiento se redujo la dimensionalidad del espacio de trabajo usando el método de la mínima fracción de ruido (MNF) pasando luego a identificar los endmembers usando el algoritmo de crecimiento de un simplex (SGA) y una vez identificados elementos presentes en las imágenes al compararlos con espectros de reflectancia obtenidos de la librería espectral USGS (United States Geological Survey), se encontraron las abundancias a través de un análisis subpixel usando el modelo de mezcla lineal (MML). En el segundo procedimiento para encontrar la abundancia de plástico presente en una imagen hiperespectral se redujo la dimensionalidad aplicando el método de análisis por componentes principales (PCA), trabajando luego en regiones de interés (ROI) de la imagen que registra el menor ruido obtenida por PCA. Estas regiones de interés permitieron obtener los espectros de reflectancia de los elementos presentes en la imagen para encontrar la matriz de transformación para el análisis de la imagen completa. Se aplicaron luego modelos de predicción de los porcentajes de granos y plásticos presentes en la imagen analizada usando el modelo PLS-DA. Estos cálculos fueron hechos usando el software libre R. Se obtuvieron también mapas de identificación de las abundancias de granos y plásticos. Los resultados muestran que el modelo PLS-SA es más eficiente que el modelo MML.
Asimismo, se identificó la presencia de micro plásticos mezclados con harina de almendra presentes en una imagen hiperespectral. Para esto se usó solamente el primer procedimiento de los que siguió en la identificación de plásticos en imágenes de mezclas de plásticos con granos, debido a que no era posible encontrar ROI’s en estas imágenes. Los resultados muestran una muy buena identificación de los plásticos presentes en granos, así como de micro plásticos presentes en harina de almendra con un error de alrededor del 2 %. Hyperspectral images of plastics mixed with almond, lentil, pea and rice grains as well as microplastics mixed with almond flour obtained in the laboratory using a hyperspectral camera RESONON model Pika NIR are presented. The identification and quantification of abundances of plastics within a group of grains present in a hyperspectral image was carried out using two ways or procedures. In the first procedure, the dimensionality of the workspace was reduced using the Minimum Noise Fraction (MNF) method, then going on to identify the endmembers using the Simplex Growing Algorithm (SGA) and once the elements pre¬sent in the images had been identified when compared with reflectance spectra obtained from the USGS spectral library (United States Geological Survey), the abundances were found th- rough a subpixel analysis using the linear mixture model (MML). In the second procedure to find the abundance of plastic present in a hyperspectral image, the dimensionality was redu- ced by applying the principal component analysis (PCA) method, then working on regions of interest (ROI) of the image that registered the least noise obtained by PCA. These regions of interest made it possible to obtain the reflectance spectra of the elements present in the image to find the transformation matrix for the analysis of the complete image. Prediction models of the percentages of grains and plastics present in the analyzed image were then applied using the PLS-DA model. For this part of the process, routines were carried out in R. Identification maps of microplastics and almond flour were also elaborated. Microplastics abundance identification maps and almond flour were also prepared. Likewise, the presence of microplastics mixed with almond flour present in a hyperspectral image was identified. For this only the the first procedure of those that were followed in the identification of plastics in images of mixtures of plastics with grains was used because it was not possible to find ROI’s in this image. The results show a very good identification of plastics present in grains as well as microplastics present in almond flour with an error of around 2 %. |
URI: | http://hdl.handle.net/20.500.14076/26993 |
Rights: | info:eu-repo/semantics/openAccess |
Appears in Collections: | Maestría |
Files in This Item:
File | Description | Size | Format | |
---|---|---|---|---|
apaza_mh.pdf | 8,53 MB | Adobe PDF | View/Open | |
apaza_mh(acta).pdf | 384,3 kB | Adobe PDF | View/Open | |
carta_de_autorización.pdf | 242,52 kB | Adobe PDF | View/Open | |
informe_de_similitud.pdf | 488,46 kB | Adobe PDF | View/Open |
This item is licensed under a Creative Commons License
Indexado por:
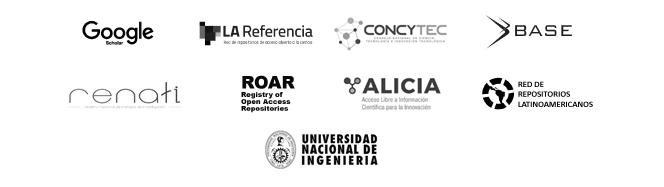