Please use this identifier to cite or link to this item:
http://hdl.handle.net/20.500.14076/1132
Title: | Predicción de la respuesta sísmica de muros de albañilería confinada empleando redes neuronales artificiales |
Authors: | Damián Jara, Melquiades Dámaso |
Advisors: | Zavala Toledo, Carlos Alberto |
Keywords: | Red Neuronal Artificial (RNA);Muro de albañilería;Red Neuronal feedforward |
Issue Date: | 2013 |
Publisher: | Universidad Nacional de Ingeniería |
Abstract: | En la presente tesis se emplea una Red Neuronal Artificial (RNA) para determinar la respuesta a una acción/desplazamiento lateral en muros de albañilería confinada. Para ello se preparan los datos de entrada al modelo, correspondiente al desplazamiento lateral y la carga vertical del muro confinado; así como la información de salida de la red, modelando el agrietamiento del muro como una secuencia de ceros y unos (0: no agrietado, 1: agrietado), además de la respuesta/fuerza lateral del muro que corresponde al patrón de agrietamiento.
Luego se diseña la arquitectura; una Red Neuronal feedforward con propagación del
error hacia atrás (Backpropagation), un algoritmo de entrenamiento de tipo gradiente descendente con momento y aprendizaje variable, y una capa oculta con 33140 neuronas.
La red se entrena para aprender los agrietamientos y las fuerzas laterales, logrando que reproduzca los datos aprendidos con aceptable precisión. In this thesis, it is employed an Artificial Neural Network (ANN) to determine the response to a lateral force/displacement in confined masonry walls. For that, the model input data are prepared, corresponding to the lateral displacement and the confined wall vertical load as well as the network output, modeling the confined wall cracking as a sequence of zeros and ones (0: non cracked, 1: cracked), besides the wall lateral response/force that corresponds to the cracking pattern. Afterwards, it is designed the architecture, a feedforward Neural Network with Backpropagation algorithm, a gradient descent training algorithm with variable moment and learning rate, and a hidden layer of 33140 neurons. The network is trained to learn the cracks and lateral forces, by making it reproduces the learnt data with acceptable precision. |
URI: | http://hdl.handle.net/20.500.14076/1132 |
Rights: | info:eu-repo/semantics/restrictedAccess |
Appears in Collections: | Maestría |
Files in This Item:
File | Description | Size | Format | |
---|---|---|---|---|
damian_jm.pdf | 2,19 MB | Adobe PDF | View/Open |
This item is licensed under a Creative Commons License
Indexado por:
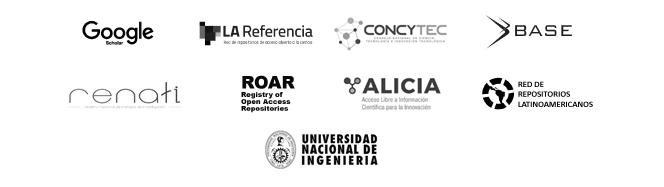